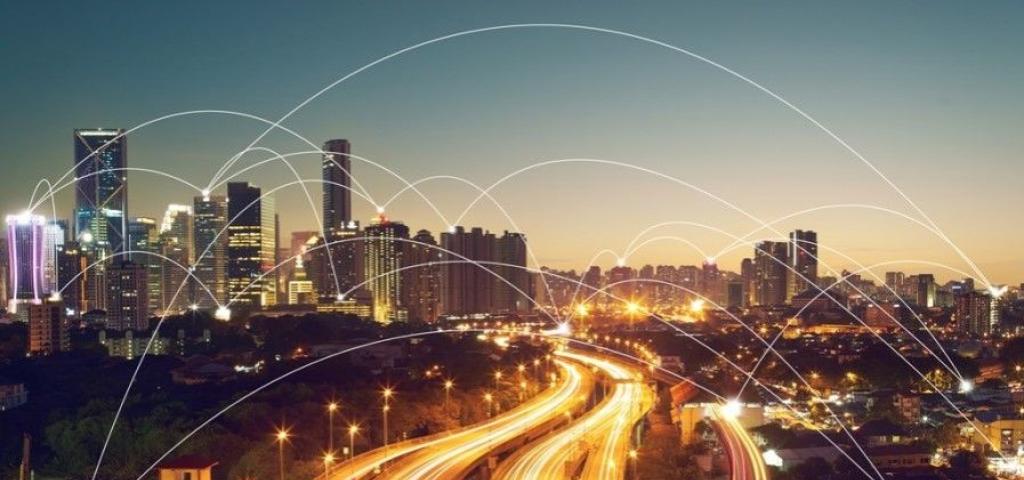
Dynamic placement optimization in edge computing scenarios can enable significant capex and opex savings, new research indicate
Guest contributed post from Arctos Labs
Edge computing is proposed to support the evolution of digital services such as live video processing, 5G, artificial intelligence, virtual reality, robotics, IoT, and direct monitoring and control of cyber-physical systems, as these applications are assumed to place higher requirements on the digital infrastructure in terms of latency, privacy, and capacity for high-performance computation.
Edge computing typically reduces the amount of data sent upwards in networks thereby contributing to less energy being consumed. There is however a risk that smaller, distributed, and heterogeneous edge infrastructure will come with lower and varying utilization, leading to lower efficiency.
To better understand these topics, Arctos Labs, Wind River and RISE (Research Institutes of Sweden) has conducted research with the aim of better understanding:
Where is the most resource-efficient location to place a specific workload at a particular time in a dynamic edge-to-cloud continuum with a fleet of edge locations?
Resource efficiency is intended to be understood in the broader meaning including energy efficiency and environmental footprint. It relates to two aspects:
1) the optimal placement of a task such that the fleet of edge and cloud infrastructure complete the computational work for the least energy at any given time, and
2) the investment decision and design of an edge-cloud data centre infrastructure related to its capacity
The research work developed a model that considers the aggregated cost for all workloads on data centre nodes (cloud and edge) in the network as well as the energy consumed by transporting data. Instead of using a simple PUE factor for each node independent of how much workload it carries, energy efficiency now depends on utilization.
The model captures that an edge data centre node has an operational sweet spot, where the overall data centre efficiency is at its best when the computational load is at around 60 – 80% utilization. This is due to that cooling and computation efficiency are not proportional to the computational work carried out. This depends on server types and their configurations, as well as ambient temperatures. The model used in the research assumed the larger cloud data centre to operate constantly close to its sweet spot.
Experiments with the model aimed to illustrate how computational load can be redistributed to the edge to bring its hardware closer to the sweet spot by comparing local and global optimization strategies over a fleet of hundreds of edge data centre nodes and an (elastic) cloud data centre.
The experiments used two types of workloads; those that must be deployed in edge DCs, and those that can be flexibly placed. Workload requests arrived with a certain intensity. The research compared two placement strategies: The baseline of placing at the edge only those workloads that must be at the edge, and all other workloads are placed in the central cloud. The second strategy placed also “flexible workloads” at the edge to bring the edge data centres to their operational sweet spot, thus operating at increased efficiency.
Results: The local and global optimization strategies that place more of the flexible workload at the edge show
(a) 4 – 6% of operational energy savings
this is due to net savings across both cloud and edge nodes due to better utilization of servers now running closer to the operational sweet spot.
(b) 50% average reduction in cloud DC hardware requirements
The hardware savings (b) are possible in the cloud DC since less of its computational resources are needed. For our simulated example of 100 edge data centre nodes with 24 servers per node, cloud capacity requirements dropped from 840 to 360 servers.
Access the research summary report here
Remark:
There are different ways by which energy consumed for data transport can be included in these types of simulations. The results above are based on “marginal increase”, i.e. the additional energy consumed by sending a certain amount of data. As fixed data transport networks have very low energy proportionality (energy consumption over the amount of data transported), the contribution from energy transport is low it is not impacting the optimal placement very much.
If we however view the issue on a more macro consumption level, the aspect of reducing the future expansion of data network capacity, from the expected data tsunami, by utilizing edge computing will have a much larger impact on the optimal placement favouring placement at the edge.
The project was funded by Datacenter Innovation Region – Tillväxt och Export at Luleå University of Technology LTU, https://datacenterinnovationregion.se