The McKinsey Simplification Model: 3 ways to incorporate robots in the automation continuum
Advanced sensor technologies, more potent computing power, and edge processing provide robots with AI capabilities. To facilitate more adoption, value, and further growth, McKinsey has developed a model15 that synthesizes industry recommendations into a single concept — simplification in three essential areas:
1. Simpler to Apply
Robot developers and integrators need to make it easier for potential end users to envision compelling scenarios. Simplification in this realm could mean something as basic as providing software that closes the gap between conceivability and installation, helping end users prove their design concepts before committing to a final investment. A prime example comes from ABB Robotics:
Visitors to the company’s website are given access to a build-your-own cobot application. Working with intuitive menus, users can browse for functions they need, with options including part handling, screwdriving, visual inspection, and “tell us more.” Users go on to select how the cobot picks up parts and puts them down; where its vision sensors are placed; what communications protocols are used; and whether the cobot will be mounted on a wall, table, or ceiling. Illustrations clarify the choices throughout. Once completed, the program evaluates the selections, then delivers a customized video simulation of how the cobot, fully installed, would perform.
2. Simpler to Connect
McKinsey advises that robot manufacturers need to deliver secure, flexible connectivity. A key goal is to achieve interoperability. The robots should be able to readily connect not only with other robots but also with the full range of intelligent systems, edge, cloud, analytics, and similar tools and devices.
Cobots rely on multiple sensors and tools such as AI to make sense of and operate safely in the world around them. Simultaneously, the environment it is installed in or traveling through will feature multiple sensor-intensive intelligent devices. The challenge is that IoT and robotics technology are often considered separate fields.16 Thus the synergies across the two disciplines go unexplored. But reimagined together, IoT and industrial robotics become the Internet of Robotic Things, or IoRT.
To date, robotics and IoT have been driven by varying yet highly related objectives. IoT focuses on supporting services for pervasive sensing, monitoring, and tracking, while the robotics community focuses on production, action, interaction, and autonomous behavior. By fusing the two fields, the resulting wider-scale digital presence means intelligent sensor and data analytics are feeding better situational awareness information to robots, which means they can better execute their tasks. In short, the robots have access to more data for analysis and decision-making. Then edge computing opens the door for even more intimate collaborations between machines and between man and machine.17
3. Simpler to Run
Paradoxically, as robots become ever more sophisticated, capable, and flexible, the effort required by end users to train them often declines. Leading manufacturers understand that shortening the learning cycle is an important means of elevating the appeal of industrial robots:
“Fanuc wants to make robots easier to train, therefore making automation more accessible to more industries,” observes TechCrunch reporter Catherine Shu.18 Accordingly, the company is harnessing AI and related technologies to accelerate teaching and learning processes. Similarly, Locus Robotics advertises warehouse robots that are so easy to train they can be deployed in just four weeks. Interfaces and tools that drive robotic learning are becoming simpler, clearer, and more efficient for end users. Such improvements are a key focus across the industry.
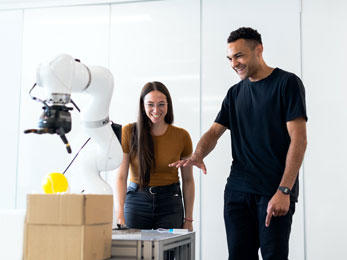